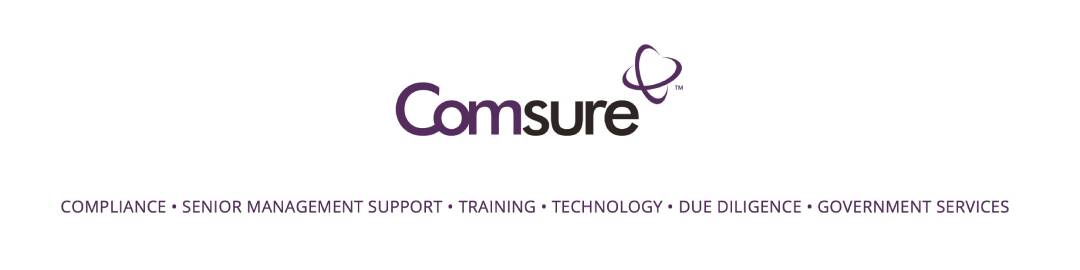
Wolfsberg Principles for Using Artificial Intelligence and Machine Learning in Financial Crime Compliance
07/03/2024
Artificial Intelligence and Machine Learning (AI/ML) can have, and are already having, a significant impact on improving the effectiveness and efficiency of financial crime compliance and risk management programmes.
The Wolfsberg Group supports the leveraging of AI/ML by Financial Institutions to detect, investigate, and manage the risk of financial crime, if appropriate data ethics principles inform the use of these technologies to ensure fair, effective, and explainable outcomes.
For this reason, the Group has published [2022-12-01] its Principles for Using Artificial Intelligence and Machine Learning in Financial Crime Compliance.
The document identifies five elements that support an ethical and responsible use of AI/ML: i. Legitimate Purpose; ii. Proportionate Use; Design and Technical Expertise; iii. Accountability and Oversight; iv. Openness and v. Transparency.
ALSO READ BELOW
Wolfsberg Principles for Using Artificial Intelligence and Machine Learning in Financial Crime Compliance
Introduction
- The Wolfsberg Group (the Group) supports the use of Artificial Intelligence and Machine Learning (AI/ML)1 by financial institutions (FIs) in their financial crime compliance programmes and believes that it is critical for FIs to consider data ethics principles when using these technologies.
- By leveraging the advances in data science underpinning AI/ML, FIs can holistically analyse the customer and transactional data created by their products and services more effectively and efficiently to detect, investigate, and manage the risk of financial crime, and satisfy regulatory requirements. By identifying potential criminal activity more effectively, FIs can focus financial crime control activities with increased precision on the customers and transactions presenting the highest risk, reducing manual reviews and customer friction such as transaction delays or redundant inquiries.
- These technology solutions, however, may require FIs to consolidate and process large amounts of data, from multiple sources.
- As a result, FIs should understand the potential impact of the use of these technologies before implementation to ensure that it results in fair, effective, and explainable outcomes. FIs should also monitor the use of AI/ML for consistent and stable performance after implementation.
- Based on the extensive regulatory, industry and academic resources existing on data ethics, 2 the Group has developed a set of principles (the principles) to guide FIs and their financial crime compliance leaders and risk management teams in identifying and managing the operational and reputational risks that may arise from the use of AI/ML.
- The principles should be operationalised by each FI according to a risk-based approach dependent on the prevailing and evolving regulatory landscape, as well as on its use of AI/ML against financial crime, and governed accordingly.
- The Wolfsberg Principles for Responsible AI/ML The Principles consist of five elements that support an FI’s responsible use of AI/ML in financial crime compliance applications.
Legitimate Purpose:
- FIs’ programmes to combat financial crimes are anchored in regulatory requirements, and a commitment to help safeguard the integrity of the financial system, while reaching fair and effective outcomes.
- Responsible use of advanced technologies such as AI/ML, and the volume and type of data necessary for them to be effective, requires FIs to understand and guard against the potential for misuse or misrepresentation of data, and any bias that may affect the results of the AI/ML application.
- A key consideration for FIs implementing AI/ML is how to integrate an assessment of ethical and operational risks into their risk governance approach.
- Moreover, the data used in AI/ML solutions adopted for the legitimate purpose of financial crimes compliance should not be allowed to support other activities without additional review under the FI’s data and risk management framework.
- In so doing, FIs will support the appropriate use of technology, which can also serve to enhance the integrity of the financial system.
Proportionate Use:
- FIs should ensure that, in their development and use of AI/ML solutions for financial crimes compliance, they are balancing the benefits of use with appropriate management of the risks that may arise from these technologies.
- Additionally, the severity of potential financial crimes risk should be appropriately assessed against any AI/ML solutions’ margin for error.
- FIs should implement a programme that validates the use and configuration of AI/ML regularly, which will help ensure that the use of data is proportionate to the legitimate, and intended, financial crimes compliance purpose.
Design and Technical Expertise:
- FIs should carefully control the technology they rely on and understand the implications, limitations, and consequences of its use to avoid ineffective financial crime risk management.
- Teams involved in the creation, monitoring, and control of AI/ML should be composed of staff with the appropriate skills and diverse experiences needed to identify bias in the results.
- Design of AI/ML systems should be driven by a clear definition of the intended outcomes and ensure that results can be adequately explained or proven given the data inputs.
- Senior stakeholders within the FI should have sufficient information on, and understanding of, AI/ML tools and their risks and benefits to make informed decisions on when, and how, such technologies will be used.
- FIs should incorporate a well-designed programme of ongoing testing, validation, and re-configuration to review AI/ML outcomes based on the intended purpose and these Principles.
Accountability and Oversight:
- FIs are responsible for their use of AI/ML, including for decisions that rely on AI/ML analysis, regardless of whether the AI/ML systems are developed in-house or sourced externally.
- FIs should train staff on the appropriate use of these technologies and consider oversight of their design and technical teams by persons with specific responsibility for the ethical use of data in AI/ML, which may be through existing risk or data management frameworks.
- FIs should also establish processes to challenge their technical teams whenever necessary and probe the use of data within their organisations.
Openness and Transparency:
- FIs should be open and transparent about their use of AI/ML, consistent with legal and regulatory requirements.
- However, care should be taken to ensure that this transparency does not facilitate evasion of the industry’s financial crime capabilities, or breach reporting confidentiality requirements and/or other data protection obligations inadvertently.
- FIs should consider engaging with regulators and educating customers about the risks and benefits of using AI/ML to prevent and detect financial crime.
Conclusion
- When considering the adoption of AI/ML solutions to address financial crime and risk management challenges, FIs should consider how their use of AI/ML aligns with their organisation’s core values, in addition to regulatory requirements.
- Existing risk management and controls structures should be adapted and expanded to ensure that any operational and reputational risks created by AI/ML are identified and managed as part of their overall risk management approach.
- AI/ML solutions can improve the effectiveness and efficiency of the detection, investigation, and management of financial crime, but ethical concerns around the use of AI/ML to manage financial crime risk should be considered and addressed.
SOURCE
NOTES
1 “AI is the science of mimicking human thinking abilities to perform tasks that typically require human intelligence, such as recognizing patterns, making predictions, recommendations, or decisions. AI uses advanced computational techniques to obtain insights from different types, sources, and quality (structured and unstructured) of data intelligence to “autonomously” solve problems and execute tasks. There are several types of AI, which operate with (and achieve) different levels of autonomy, but in general, AI systems combine intentionality, intelligence, and adaptability”; “Machine Learning is a type (subset) of AI that “trains” computer systems to learn from data, identify patterns and make decisions with minimal human intervention. Machine learning involves designing a sequence of actions to solve a problem automatically through experience and evolving pattern recognition algorithms with limited or no human intervention — i.e., it is a method of data analysis that automates analytical model building. Respondents cite machine learning and natural language processing as the AI-powered capabilities offering great benefit to AML/CFT for regulated entities and supervisors. Machine learning reportedly offers the greatest advantage through its ability to learn from existing systems, reducing the need for manual input into monitoring, reducing false positives and identifying complex cases, as well as facilitating risk management." Opportunities and Challenges of New Technologies for AML/CFT, Financial Action Task Force - fatf-gafi.org (July 2021)
2 See e.g. The OECD Artificial Intelligence (AI) Principles - OECD.AI (May 2019); Statement of Kevin Greenfield, Deputy Comptroller for Operational Risk Policy before the Task Force on Artificial Intelligence, Committee on Financial Services, U.S. House of Representatives - OCC.gov (May 2022); Guidance on the Ethical Development and Use of Artificial Intelligence, Office of the Privacy Commissioner for Personal Data, Hong Kong - PCPD.org.hk (August 2021); White Paper on Artificial Intelligence: a European approach to excellence and trust, European Commission - (europa.eu) (February 2020); Principles to Promote Fairness, Ethics, Accountability and Transparency (FEAT) in the Use of Artificial Intelligence and Data Analytics in Singapore’s Financial Sector, Monetary Authority of Singapore - mas.gov.sg (November 2018).
SOURCE
The Team
Meet the team of industry experts behind Comsure
Find out moreLatest News
Keep up to date with the very latest news from Comsure
Find out moreGallery
View our latest imagery from our news and work
Find out moreContact
Think we can help you and your business? Chat to us today
Get In TouchNews Disclaimer
As well as owning and publishing Comsure's copyrighted works, Comsure wishes to use the copyright-protected works of others. To do so, Comsure is applying for exemptions in the UK copyright law. There are certain very specific situations where Comsure is permitted to do so without seeking permission from the owner. These exemptions are in the copyright sections of the Copyright, Designs and Patents Act 1988 (as amended)[www.gov.UK/government/publications/copyright-acts-and-related-laws]. Many situations allow for Comsure to apply for exemptions. These include 1] Non-commercial research and private study, 2] Criticism, review and reporting of current events, 3] the copying of works in any medium as long as the use is to illustrate a point. 4] no posting is for commercial purposes [payment]. (for a full list of exemptions, please read here www.gov.uk/guidance/exceptions-to-copyright]. Concerning the exceptions, Comsure will acknowledge the work of the source author by providing a link to the source material. Comsure claims no ownership of non-Comsure content. The non-Comsure articles posted on the Comsure website are deemed important, relevant, and newsworthy to a Comsure audience (e.g. regulated financial services and professional firms [DNFSBs]). Comsure does not wish to take any credit for the publication, and the publication can be read in full in its original form if you click the articles link that always accompanies the news item. Also, Comsure does not seek any payment for highlighting these important articles. If you want any article removed, Comsure will automatically do so on a reasonable request if you email info@comsuregroup.com.